Elements of statistical data processing. Statistical data processing and its features Consolidation of the studied material
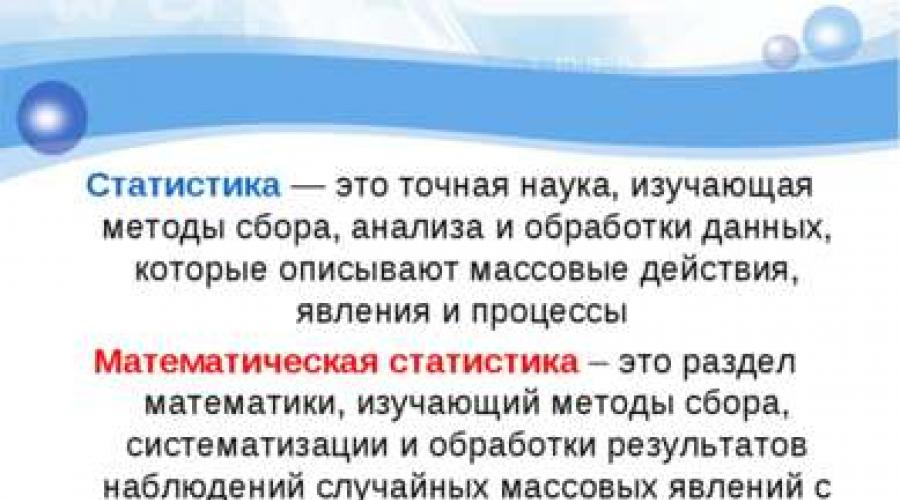
Read also
slide 1
slide 2

slide 3

slide 4

slide 5

slide 6

Slide 7

Slide 8

Slide 9

slide 10

slide 11

slide 12

slide 13

slide 14

slide 15

slide 16

slide 17

slide 18

slide 19

slide 20

slide 21

slide 22

slide 23

slide 24

slide 25

slide 26

slide 27

slide 28

slide 29

The methods of statistical processing of the results of the experiment are mathematical methods, formulas, methods of quantitative calculations, with the help of which the indicators obtained during the experiment can be generalized, brought into the system, revealing the patterns hidden in them.
We are talking about such regularities of a statistical nature that exist between the variables studied in the experiment.
Data are the main elements to be classified or categorized for the purpose of processing 26 .
Some of the methods of mathematical and statistical analysis make it possible to calculate the so-called elementary mathematical statistics that characterize the sample distribution of data, for example:
sample mean,
Sample variance,
Median and others.
Other methods of mathematical statistics make it possible to judge the dynamics of changes in individual sample statistics, for example:
dispersion analysis,
Regression analysis.
Using the third group of sampling methods, one can reliably judge the statistical relationships that exist between the variables that are examined in this experiment:
Correlation analysis;
Factor analysis;
comparison methods.
All methods of mathematical-statistical analysis are conventionally divided into primary and secondary 27 .
Methods are called primary, with the help of which it is possible to obtain indicators that directly reflect the results of measurements made in the experiment.
Secondary methods are called statistical processing, with the help of which, on the basis of primary data, statistical patterns hidden in them are revealed.
Primary statistical processing methods include, for example:
Determination of the sample mean;
Sample variance;
Selective fashion;
Sample median.
Secondary methods typically include:
Correlation analysis;
Regression analysis;
Methods for comparing primary statistics for two or more samples.
Let's consider methods for calculating elementary mathematical statistics, starting with the sample mean.
Arithmetic mean - is the ratio of the sum of all data values to the number of terms 28 .
The average value as a statistical indicator is the average assessment of the psychological quality studied in the experiment.
This assessment characterizes the degree of its development as a whole in the group of subjects that was subjected to a psychodiagnostic examination. Comparing directly the average values of two or more samples, we can judge the relative degree of development in the people who make up these samples of the quality being assessed.
The sample mean is determined using the following formula 29:
where x cf is the sample mean or arithmetic mean of the sample;
n - the number of subjects in the sample or private psychodiagnostic indicators, on the basis of which the average value is calculated;
x k - private values of indicators for individual subjects. There are n such indicators, so the index k of this variable takes values from 1 to n;
∑ - accepted in mathematics, the summation sign of the values of those variables that are to the right of this sign.
Dispersion is a measure of the scatter of the data about the mean value of 30 .
The greater the variance, the greater the variance or scatter in the data. It is determined in order to be able to distinguish from each other quantities that have the same average, but different spread.
The dispersion is determined by the following formula:
where is the sample variance, or simply the variance;
An expression meaning that for all x k from the first to the last in this sample, it is necessary to calculate the differences between private and average values, square these differences and sum them up;
n is the number of subjects in the sample or primary values for which the variance is calculated.
median the value of the trait under study is called, which divides the sample, ordered by the value of this trait, in half.
Knowing the median is useful in order to establish whether the distribution of particular values of the studied trait is symmetrical and approaches the so-called normal distribution. The mean and median for a normal distribution are usually the same or differ very little from each other.
If the sample distribution of features is normal, then secondary statistical calculation methods based on the normal distribution of data can be applied to it. Otherwise, this cannot be done, since serious errors can creep into the calculations.
Fashion one more elementary mathematical statistics and characteristic of distribution of experimental data. Mode is the quantitative value of the trait under study, which is most often found in the sample.
For symmetric feature distributions, including the normal distribution, the mode values coincide with the mean and median values. For other types of distributions, asymmetric, this is not typical.
The method of secondary statistical processing, through which the relationship or direct relationship between two series of experimental data is found out, is called correlation analysis method. It shows how one phenomenon affects another or is related to it in its dynamics. Dependencies of this kind exist, for example, between quantities that are in causal relationships with each other. If it turns out that two phenomena are statistically significantly correlated with each other, and if at the same time there is confidence that one of them can act as a cause of the other phenomenon, then it definitely follows that there is a causal relationship between them.
There are several varieties of this method:
Linear correlation analysis allows you to establish direct links between variables in their absolute values. These connections are graphically expressed by a straight line, hence the name "linear".
The linear correlation coefficient is determined using the following formula 31:
where r xy - linear correlation coefficient;
x, y - average sample values of compared values;
X i ,y i - private sample values of compared quantities;
P - the total number of values in the compared series of indicators;
Dispersions, deviations of compared values from average values.
Rank correlation determines the dependence not between the absolute values of variables, but between ordinal places, or ranks, occupied by them in a series ordered by magnitude. The formula for the rank correlation coefficient is 32:
where R s - coefficient of rank correlation according to Spearman;
d i - the difference between the ranks of indicators of the same subjects in ordered rows;
P - the number of subjects or digital data (ranks) in the correlated series.
Laboratory work №3. Statistical data processing in the MatLab system
General statement of the problem
The main purpose of the implementation laboratory work is to get acquainted with the basics of working with statistical data processing in the MatLAB environment.
Theoretical part
Primary statistical data processing
Statistical processing of data is based on primary and secondary quantitative methods. The purpose of the primary processing of statistical data is to structure the information received, which implies grouping the data into pivot tables according to various parameters. Raw data should be presented in such a format that a person can make an approximate assessment of the received data set and reveal information about the data distribution of the received data sample, for example, the homogeneity or compactness of the data. After the primary data analysis, methods of secondary statistical data processing are applied, on the basis of which statistical patterns are determined in the existing data set.
Conducting a primary statistical analysis on a data array allows you to gain knowledge about the following:
What is the most typical value for the sample? For an answer to this question measures of the central tendency are determined.
Is there a large scatter of data relative to this characteristic value, i.e., what is the “fuzziness” of the data? In this case, measures of variability are determined.
It is worth noting the fact that the statistical indicators of the measure of central tendency and variability are determined only on quantitative data.
Measures of central tendency- a group of values around which the rest of the data are grouped. Thus, the measures of the central tendency generalize the data array, which makes it possible to form inferences both about the sample as a whole and to conduct comparative analysis different samples with each other.
Suppose there is a data sample , then the measures of the central tendency are estimated by the following indicators:
1. sample mean is the result of dividing the sum of all sample values by their number. It is determined by formula (3.1).
(3.1)
Where - i-th sample element;
n is the number of sample elements.
The sample mean provides the greatest accuracy in the process of estimating the central trend.
Let's say we have a sample of 20 people. Sample elements are information about the average monthly income of each person. Suppose that 19 people have an average monthly income of 20k. and 1 person with an income of 300 tr. The total monthly income of the entire sample is 680 tr. The sample mean in this case is S=34.
2. Median- generates a value above and below which the number of different values is the same, i.e. this is the central value in a sequential data series. It is determined depending on the evenness / oddness of the number of elements in the sample using formulas (3.2) or (3.3). Algorithm for estimating the median for a data sample:
First of all, the data is ranked (ordered) in ascending/descending order.
If the ordered sample has an odd number of elements, then the median is the same as the center value.
(3.2)
Where n
In the case of an even number of elements, the median is defined as the arithmetic mean of the two central values.
(3.3)
where is the average element of the ordered sample;
- element of ordered selection following ;
The number of sample elements.
In the event that all the elements of the sample are different, then exactly half of the elements of the sample are greater than the median, and the other half are less. For example, for the sample (1, 5, 9, 15, 16), the median is the same as element 9.
In statistical data analysis, the median allows you to identify the elements of the sample that strongly affect the value of the sample mean.
Let's say we have a sample of 20 people. Sample elements are information about the average monthly income of each person. Suppose that 19 people have an average monthly income of 20k. and 1 person with an income of 300 tr. The total monthly income of the entire sample is 680 tr. The median, after ordering the sample, is defined as the arithmetic mean of the tenth and eleventh elements of the sample) and is equal to Me = 20 tr. This result is interpreted as follows: the median divides the sample into two groups, so that it can be concluded that in the first group each person has an average monthly income of no more than 20 thousand rubles, and in the second group no less than 20 thousand rubles. IN this example we can say that the median is characterized by how much the “average” person earns. While the value of the sample average is significantly higher than S=34, which indicates the unacceptability of this characteristic when assessing average earnings.
Thus, the greater the difference between the median and the sample mean, the greater the scatter of the sample data (in the considered example, a person with an earnings of 300 tr. is clearly different from the average people in a particular sample and has a significant impact on the average income estimate). What to do with such elements is decided in each individual case. But in the general case, to ensure the reliability of the sample, they are withdrawn, since they have a strong influence on the assessment of statistical indicators.
3. Fashion (Mo)- generates the value that occurs most frequently in the sample, i.e. the value with the highest frequency. Mode estimation algorithm:
In the case when the sample contains elements that occur equally often, then we say that there is no mode in such a sample.
If two adjacent bins have the same frequency, which is greater than the frequency of the other bins, then the mode is defined as the average of the two values.
If two elements of the sample have the same frequency, which is greater than the frequency of the remaining elements of the sample, and at the same time these elements are not adjacent, then we say that there are two modes in this sample.
Mode in statistical analysis is used in situations where it is necessary to quickly estimate the measure of central tendency and high accuracy is not required. For example, fashion (in terms of size or brand) is convenient to use to determine the clothes and shoes that are most in demand among buyers.
Measures of scatter (variability)- a group of statistical indicators characterizing the differences between separate values samples. Based on the indicators of dispersion measures, it is possible to assess the degree of homogeneity and compactness of the sample elements. Scatter measures are characterized by the following set of indicators:
1. Swipe - this is the interval between the maximum and minimum values of the results of observations (sample elements). The range indicator indicates the spread of values in a dataset. If the range is large, then the values in the population are very scattered, otherwise (the range is small), it is said that the values in the population lie close to each other. The range is determined by formula (3.4).
(3.4)
Where - the maximum element of the sample;
is the minimum element of the sample.
2.Average deviation is the arithmetic mean difference (in absolute value) between each value in the sample and its sample mean. The average deviation is determined by formula (3.5).
(3.5)
Where - i-th sample element;
The value of the sample mean, calculated by formula (3.1);
The number of sample elements.
Module necessary due to the fact that deviations from the average for each specific element can be both positive and negative. Therefore, if the modulus is not taken, then the sum of all deviations will be close to zero and it will be impossible to judge the degree of data variability (data crowding around the sample mean). In statistical analysis, the mode and median may be taken instead of the sample mean.
3. Dispersion is a measure of scatter that describes the relative deviation between data values and the mean. It is calculated as the sum of the squared deviations of each sample element from the mean value. Depending on the sample size, the variance is estimated different ways:
For large samples (n>30) according to the formula (3.6)
(3.6)
For small samples (n<30) по формуле (3.7)
(3.7)
where X i - i-th element of the sample;
S is the mean value of the sample;
Number of sample elements;
(X i – S) - deviation from the mean value for each value of the data set.
4. Standard deviation is a measure of how widely scattered the data points are relative to their mean.
The process of squaring individual deviations in calculating the variance increases the degree of deviation of the obtained deviation value from the original deviations, which in turn introduces additional errors. Thus, in order to approximate the estimate of the spread of data points about their average to the value of the average deviation, the square root is extracted from the variance. The extracted root of the variance characterizes a measure of variability called the root mean square or standard deviation (3.8).
(3.8)
Let's say you're a software development project manager. You have five programmers under your supervision. By managing the process of project execution, you distribute tasks among programmers. For simplicity of the example, we will proceed from the fact that the tasks are equivalent in complexity and execution time. You decided to analyze the work of each programmer (the number of completed tasks during the week) for the last 10 weeks, as a result of which you received the following samples:
Week Name | ||||||||||
After evaluating the average number of completed tasks, you got the following result:
Week Name | S | ||||||||||
22,3 | |||||||||||
22,4 | |||||||||||
22,2 | |||||||||||
22,1 | |||||||||||
22,5 |
Based on the S indicator, all programmers, on average, work with the same efficiency (about 22 tasks per week). However, the indicator of variability (range) is very high (from 5 tasks for the fourth programmer to 24 tasks for the fifth programmer).
Week Name | S | P | ||||||||||
22,3 | ||||||||||||
22,4 | ||||||||||||
22,2 | ||||||||||||
22,1 | ||||||||||||
22,5 |
Let's estimate the standard deviation, which shows how the values are distributed in the samples relative to the mean, namely, in our case, to estimate how large the spread of task completion is from week to week.
Week Name | S | P | SO | ||||||||||
22,3 | 1,56 | ||||||||||||
22,4 | 1,8 | ||||||||||||
22,2 | 2,84 | ||||||||||||
22,1 | 1,3 | ||||||||||||
22,5 | 5,3 |
The resulting estimate of the standard deviation says the following (let's evaluate the two extreme cases 4 and 5 programmers):
Each value in a sample of 4 programmers, on average, deviates by 1.3 jobs from the mean.
Each value in the programmer's sample 5 deviates, on average, by 5.3 jobs from the mean.
The closer the standard deviation is to 0, the more reliable the mean is, as it indicates that each value in the sample is nearly equal to the mean (22.5 items in our example). Therefore, the 4th programmer is the most consistent in contrast to the 5th. The week-to-week variability of task completion for the 5th programmer is 5.3 tasks, which indicates a significant spread. In the case of the 5th programmer, the average cannot be trusted, and therefore it is difficult to predict the number of tasks completed for the next week, which in turn makes it difficult to plan and adhere to work schedules. What managerial decision you make in this course is unimportant. It is important that you receive an assessment on the basis of which appropriate management decisions can be made.
Thus, a general conclusion can be drawn that the mean does not always correctly estimate the data. The correctness of the estimate of the mean can be judged by the value of the standard deviation.
Lecture 12. Methods of statistical processing of results.
Methods of statistical processing of results are called mathematical techniques, formulas, methods of quantitative calculations, with the help of which the indicators obtained during the experiment can be generalized, brought into a system, revealing the patterns hidden in them. We are talking about such regularities of a statistical nature that exist between the variables studied in the experiment.
1. Methods of primary statistical processing of experimental results
All methods of mathematical and statistical analysis are conditionally divided into primary and secondary. Methods are called primary, with the help of which it is possible to obtain indicators that directly reflect the results of measurements made in the experiment. Accordingly, primary statistical indicators mean those that are used in the psychodiagnostic methods themselves and are the result of the initial statistical processing of the results of psychodiagnostics. Secondary methods are called statistical processing, with the help of which, on the basis of primary data, statistical patterns hidden in them are revealed.
Primary statistical processing methods include, for example, the determination of the sample mean, sample variance, sample mode, and sample median. Secondary methods usually include correlation analysis, regression analysis, methods for comparing primary statistics in two or more samples.
Consider methods for calculating elementary mathematical statistics.
Fashion called the quantitative value of the trait under study, the most common in the sample.
median the value of the trait under study is called, which divides the sample, ordered by the value of this trait, in half.
sample mean(arithmetic mean) value as a statistical indicator is the average assessment of the psychological quality studied in the experiment.
scatter(sometimes this value is called the range) of the sample is denoted by the letter R. This is the simplest indicator that can be obtained for the sample - the difference between the maximum and minimum values \u200b\u200bof this particular variation series.
Dispersion is the arithmetic mean of the squares of the deviations of the values of a variable from its mean value.
2. Methods of secondary statistical processing of experimental results
With the help of secondary methods of statistical processing of experimental data, hypotheses related to the experiment are directly verified, proved or refuted. These methods, as a rule, are more complicated than the methods of primary statistical processing, and require the researcher to be well-trained in elementary mathematics and statistics.
The discussed group of methods can be divided into several subgroups:
1 regression calculus
Regression calculus is a method of mathematical statistics that allows you to reduce private, disparate data to a certain linear graph that approximately reflects their internal relationship, and to be able to approximately estimate the probable value of another variable by the value of one of the variables.
2. Correlation
The next method of secondary statistical processing, by means of which the connection or direct dependence between two series of experimental data is found out, is called the method of correlations. It shows how one phenomenon affects another or is related to it in its dynamics. Dependencies of this kind exist, for example, between quantities that are in causal relationships with each other. If it turns out that two phenomena are statistically significantly correlated with each other, and if at the same time there is confidence that one of them can act as a cause of the other phenomenon, then it definitely follows that there is a causal relationship between them.
3 Factor analysis
Factor analysis is a statistical method that is used when processing large amounts of experimental data. The tasks of factor analysis are: reducing the number of variables (data reduction) and determining the structure of relationships between variables, i.e. classification of variables, so factor analysis is used as a data reduction method or as a structural classification method.
Review questions
1.What are statistical processing methods?
2. What subgroups are the secondary methods of statistical processing divided into?
3. Explain the essence of the correlation method?
4. In what cases are statistical processing methods used?
5. In your opinion, how effective is the use of statistical processing methods in scientific research?
2. Consider the features of statistical data processing methods.
Literature
1.. Gorbatov D.S. Workshop on psychological research: Proc. allowance. - Samara: "BAHRAKH - M", 2003. - 272 p.
2. Ermolaev A.Yu. Mathematical statistics for psychologists. - M.: Moscow Psychological and Social Institute: Flint, 2003.336s.
3. Kornilova T.V. Introduction to the psychological experiment. Textbook for universities. M.: CheRo Publishing House, 2001.